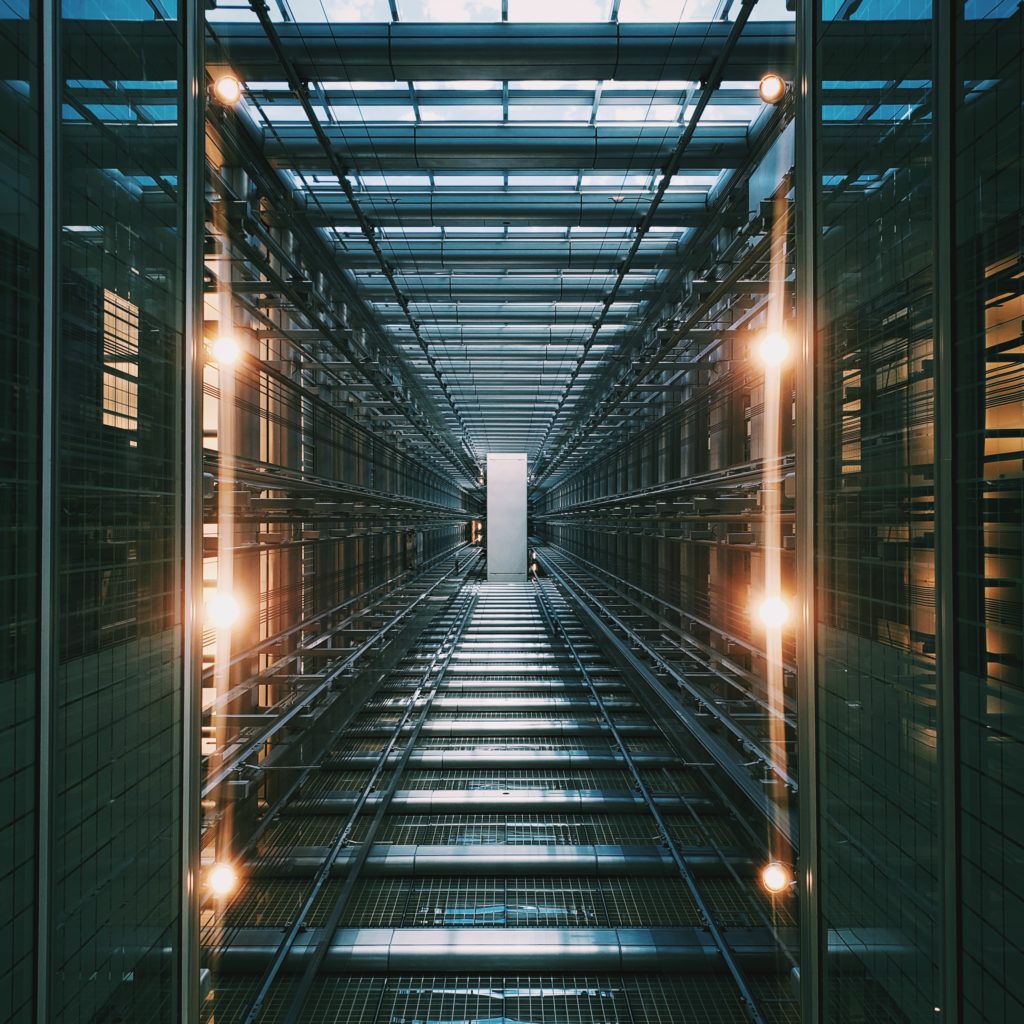
Deep Blue, Alpha Zero, HAL 9000, SkyNet, and… your reserve model? The idea that you’re facing off against a multi-billion dollar silicon-based mind capable of destroying humanity (or just winning every chess game ever) may be a little far-fetched. But a growing number of actuaries have, in the very recent past, run into artificial intelligence models which are affecting their work. And if they haven’t, they will soon. This article will give a quick introduction to some potential uses for such models, some options for exploration, and a few cautions along the way so that you don’t make a mistake leading to costly delays or distracted effort.
What are “Artificial Intelligence” and “Machine Learning”?
We’d love to start by setting baselines around terminology. Unfortunately, we won’t be able to conclusively do that, because it seems as if just about everyone has their own definitions of the concepts involved.
Artificial intelligence (AI) is a branch of data science, as is machine learning (ML). Both relate to the use of large volumes of data. Both relate to building predictive tools based on historical relationships within those data sets. And the two terms have quite a lot of overlap, depending on your criteria for differentiation.
How do they differ?
Some professionals see ML as the more fundamental, technical applications, answering questions related to precision: How can we reduce the variance in our estimate of next month’s claims? Can we add some more variables from another data set so that our pre-lapse intervention efforts are more targeted and effective? Generally, those professionals may use existing data sets or models and seek to add additional predictive power to what already exists.
They often view AI as appropriate for more strategic, what-if based applications. If we refine our investment strategy to consider new asset classes, how does it change our estimates of free capital five years out? Could we develop new underwriting criteria that would give us better coverage in the underserved low-income market? These questions may not be easily answered with traditional linear regression models, which require vast amounts of comparable experience in order to gain predictive power. But allowing a neural network to churn through all the potential interactions amongst hundreds or thousands of variables to present the entire universe of potential solutions for consideration certainly is appealing.
How are they similar?
Others see the terms interchangeably, or even all together as one: AI/ML. They will view the overlap more generously, and will try not to draw distinctions between whether an application is strictly AI or ML. They can do this because the tools and techniques (such as programming languages, data storage and retrieval, or variance reduction processes) are going to be similar regardless of the use case to which they are applied.
For the rest of this article, we’ll take the second approach, and speak of AI/ML models and applications. This should not be any indication that one perspective is preferred over the other, just that the ideas and concepts herein are more general rather than specific. And caveats and conclusions apply across both disciplines.
What can AI/ML models do?
(Photo credit: Alex Knight)
Reduce decision friction:
Traditionally, actuarial processes such as pricing cycles or reserve analyses may have resulted in a static output set: a vast array of numbers which aggregate to one or two key indicators. If there’s a question about impacts or sensitivities, that may require actuaries to go back to their models and return after some delay (2 days? 3?) with another static answer. Which adds a lot of friction to the decisions that the stakeholders are trying to make based on those values and significantly slows the process.
Jean-Philippe Larochelle, who has years of experience building and implementing AI/ML models for insurance companies with Ernst & Young, said there’s a better way.
“Many insurance companies are in the process of transforming or modernizing their actuarial processes. However, actuaries often do not realize how impactful the introduction of AI/ML capabilities can be on traditional actuarial processes.
Actuaries are looking to increase automation and their ability to generate analytical information for pricing, financial planning & analysis, asset liability management, hedging and more. When redesigning these processes, actuaries should consider strategically introducing the use of AI/ML models as it can fundamentally transform how actuaries produce their analysis.
For instance, instead of providing a static spreadsheet or PDF report, actuaries can embed AI/ML in dashboards and allow management to run various what-ifs analysis in real-time. Actuaries can also use AI/ML to speed up actuarial calculations or generate automatic experience monitoring insights”
Enable new analyses:
Principles-based reserves in the U.S. require stochastic interest rate scenarios, which alone require a lot of computing power. When performing what-if analysis or forecasting business months or years into the future, the models would be better predictors of the future if those reserves within each scenario could be further calculated using a second set of stochastic scenarios. This stochastic-on-stochastic analysis has traditionally been too computationally intense to be of any practical application. Each additional step in the calculation increases the number of calculations (and subsequent time) exponentially. If there were 1,000 initial scenarios, two layers down requires 1,000,000 scenarios. And that only supports one further step. Each additional level, Inception-like, adds another factor of 1,000.
[Not to mention the fact that under certain reporting structures, a vast majority of your work could just be wasted. When you’re looking at a CTE70 metric (conditional tail expectation at the 70th percentile), you’ve potentially done 70% of that work for zero benefit.]
However, with AI/ML techniques, perhaps a system could be trained to understand which types of scenarios are more relevant or impactful to reserves, and only produce second-level scenarios of those specific types. [Like the 30% that are relevant to your CTE metric.] Maybe the model can learn to choose a subset of 50 scenarios that have important characteristics impacting reserves for a given portfolio. Now, instead of 1,000,000 scenarios for 2 steps, perhaps it’s only 50,000, a significant reduction in processing time and data storage. This is a dramatic improvement in the potential usability of results, because of the option to make many more what-if evaluations in the same span of time (20 versus 1). It should be clear that twenty times the coverage could seriously improve the chance of including all the important risks in any analysis.
Maintaining fresh assumptions:
One problem with traditional actuarial models is that the assumptions used may be out of date by the time the actual analysis is performed, because they were set in an earlier period and subsequently the environment has changed. [COVID-19 anyone? Or how about signing an Asset Adequacy Analysis opinion in March, based on a model from September of the prior year?] Assumption-setting processes require a lot of data and time for analysis, so simplifications are made, often by holding the same set of assumptions from period to period, or maybe applying some judgment as to the importance of any suggested changes.
Economic scenario reduction:
One current application of this is in the area of selecting economic scenarios for periodic balance sheet risk evaluations. Considering different movements of interest rates (both risk-free and risk-adjusted) and equity market changes, the possibilities number well into the tens of thousands. For this example, let’s assume a company believes 1,000 possible material combinations exist. In order to speed up the process, the decision-makers choose a smaller number of 50 analytic scenarios each quarter out of the entire matrix of possibilities. This is done in order to save processing time, balance loss of information with expediency, and focus on the “most important” combinations.
But which scenarios are important this quarter? Are the 50 scenarios that were important last quarter going to be just as important this quarter? Are there other scenarios that pose potential, undisclosed risks that the company should know about? How would the company know unless it ran all 1,000 scenarios and compared the results against the reduced set? But doing so defeats the purpose of performing the scenario reduction exercise in the first place.
Again, this may be an application for AI/ML techniques: to develop a model of the model’s characteristics and interactions between the demographics of the in-force portfolio, the asset base, and the external economic environment. This meta-model can then be used to inform the decision on which scenarios should be selected, with less degradation of predictive power as time passes. This enables the model to essentially self-calibrate, ensuring that the analyses derived from it don’t lose their usefulness in the future.
Some cautions to watch out for with AI/ML models
As always, we must caution against incorrect or uninformed application of the model. Actuaries need to thoroughly test any work product if they have outsourced the development of the model or its parameters to a data science team, either within the company or an external consultant. They must validate that the training of the model was done appropriately, that the limitations and biases are understood, and that it’s not going to produce nonsensical or unusable results.
Also, watch out for modern applications that don’t play well with historic systems. Here’s Mr. Larochelle again: “As you’re modernizing your processes is really where you have the opportunity to get the most out of AI. If you’re working with older systems, maybe models that you built 10, 20 years ago, perhaps Excel-only models, and looking at implementing AI/ML, having modernized systems is going to give you a much better return. If you’re trying to just jam AI into old systems, there’s going to be a number of challenges to overcome.”
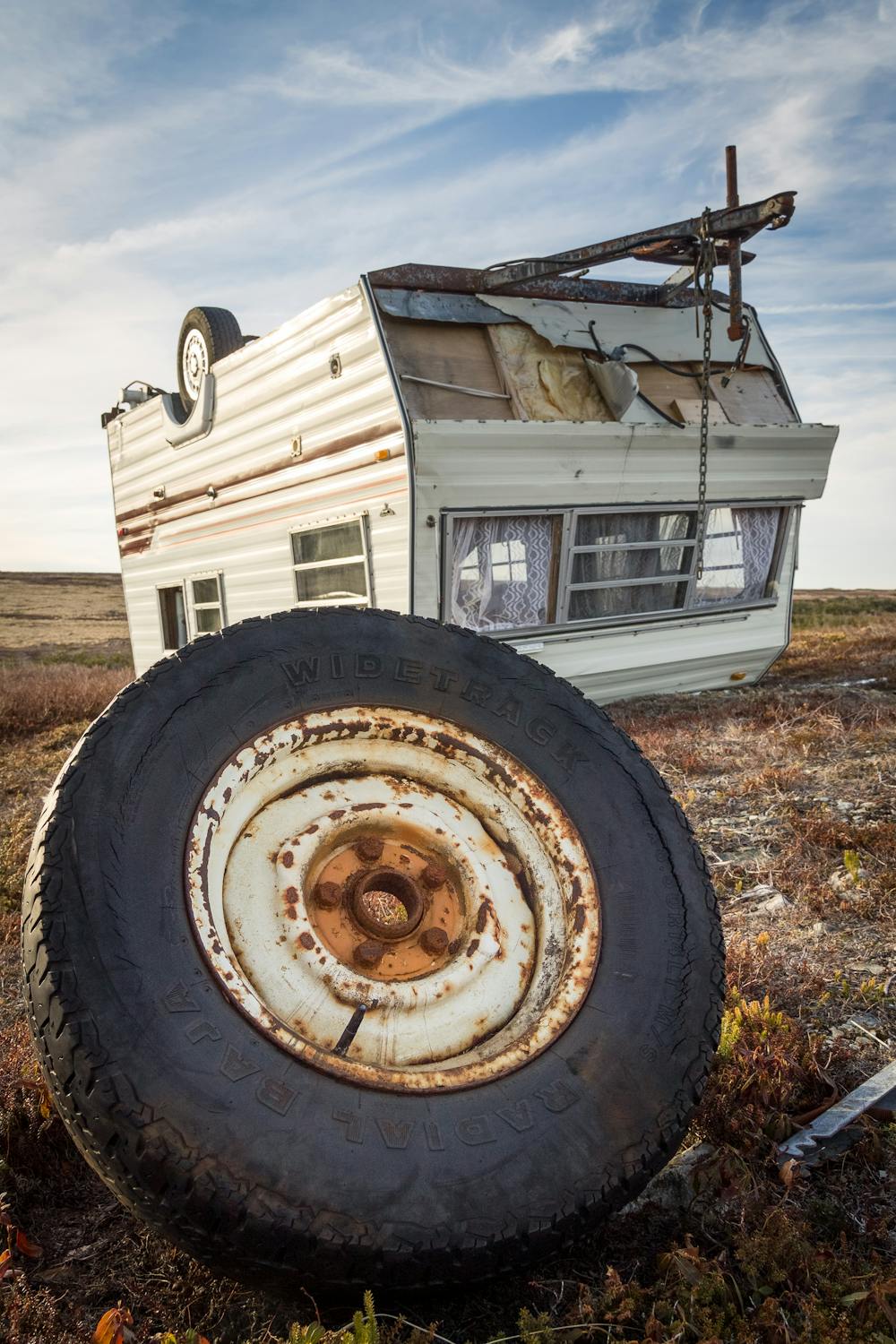
You wouldn’t hitch a Ferrari to a trailer
Basically, if you’re trying to do this modern work on an ancient infrastructure or software stack, you’ll be setting yourself up for significant headaches in terms of integration or, ironically, a loss of predictive power. This could happen if you have an AI/ML model that produces a set of outputs that don’t fit well into the traditional actuarial system. In order to conform, there may be transformations or simplifications which degrade the effectiveness of that AI/ML model. Which means you’re not really getting the value from the investment that you hoped or were promised.
And because we like the term, we must remind you to watch out for Frankenmodels – where you just stick something on to the outside of an existing system, hoping it works. This adds maintenance time and cost, allows more opportunities for error, and overall increases the headaches involved. Far better would be to have your AI/ML model integrated with your actuarial projection model, so that the various parts could talk to one another and inform each other. This is incredibly difficult to do with traditional systems, which just weren’t built for such dynamic interaction or the volume of data necessary. So once again, you may not be getting the value you were promised if the systems are not set up using modern technologies.
[Spoiler alert – Slope is working on exactly this kind of integration. Be on the lookout in the very near future for an announcement. 😉 ]
AI/ML isn’t just for actuaries, either
And don’t forget, even if you’re not directly using any kind of AI/ML in your own work, you could still see impacts from data science initiatives at points higher up in the policy life cycle. Marketing and underwriting may be applying AI/ML models that you didn’t know about to attract different customers and issue them different policies. That would show up in your experience studies and actual-to-expected results. Or perhaps the investment department is using some advanced algorithms to select different assets, which could affect future returns.
Basically, you can’t just put your head in the sand and hope that these ideas go away. Data science is here, and so it’s imperative that the actuary understands the implications, even if there are no direct applications within your company. At the very least, many in the industry are already implementing it. If you don’t want to become uncompetitive, you’ll have to get up to speed quickly.
So much to learn. Where to start?
Obviously, generic web searching will produce an overwhelming volume of material around data science and its applications. Some good blogs that may be helpful include online communities like Towards Data Science and Data Science Central.
For more actuarial-specific information, look to the Society of Actuaries: This page has an extensive list of resources. Plus the Emerging Technologies and Machine Learning Methods reports have direct applications to actuarial work.
Another great way would be to reach out to current DS professionals at your company. Start by trying to build a relationship. Understand what topics are of interest to them, and pose to them some of the questions that have been plaguing you. Is it experience studies? Or reserve analysis? Pricing cycle? We’ve discovered that having actuaries work with non-actuaries has been essential to actually developing a deep understanding of the real problems involved.
You may have no immediate business case, and that’s quite alright. Often, not being constrained by existing expectations is a good thing. During your discussions, the ideas can spontaneously flow and something may emerge as a confluence of what you need help with but couldn’t articulate, and what they can do but didn’t know anyone was struggling with.
Reminder: Remember why you’re doing this in the first place
This is going to start to sound like a tired refrain, because we’ve said it so often. [Here, here, and here, for starters.] Still, we think it’s important, so we’ll continue to point it out: Remember why you’re building models anyway.
These models, whether using AI/ML or not, exist for the benefit of the business. They’re not around just to give you an opportunity to refine your skills at R or Python.
Sure, it may be fun and exciting to nerd-out on getting your K-means factor down an additional 20%, or minimizing the volatility of the A:E in your 5-quarter forward reserve projection. But are those numerical refinements useful? Do they…
- Help your Chief Actuary become more confident that the reserves themselves are sufficient?
- Make the head of marketing better able to accept your new rates, as “uncompetitive” as she says they are, because they appropriately balance the risks to the policyholder, the agents, and the company?
- Give your CFO greater trust in your forecast of free cash flow for the next 18 months, thus enabling the go/no-go decision on that line of business expansion?
The whole point is not just numbers for numbers’ sake. The point is to make something useful. These models and applications are intended to help the business make better decisions, informed by the risk/return tradeoffs inherent in the options available.
That’s what AI/ML can do: help you be a better actuary. These models, whether using AI/ML or just the law of large numbers applied to portfolios of risks, exist to enable stakeholders to accept the risks of the options inherent in their choices. And it’s the responsibility of the actuary to understand and use those models to provide wise counsel, so those decisions can be made with the highest probability of success.
Afterword
If you and your models can’t actually do that right now, maybe it’s time for an upgrade. If it’s your skills, that’s on you. But if it’s your system, that’s where we shine. Give us a call. We’d love to show you how to integrate AI/ML tools with your actuarial projections in a cloud-based software accessible with just a web browser.