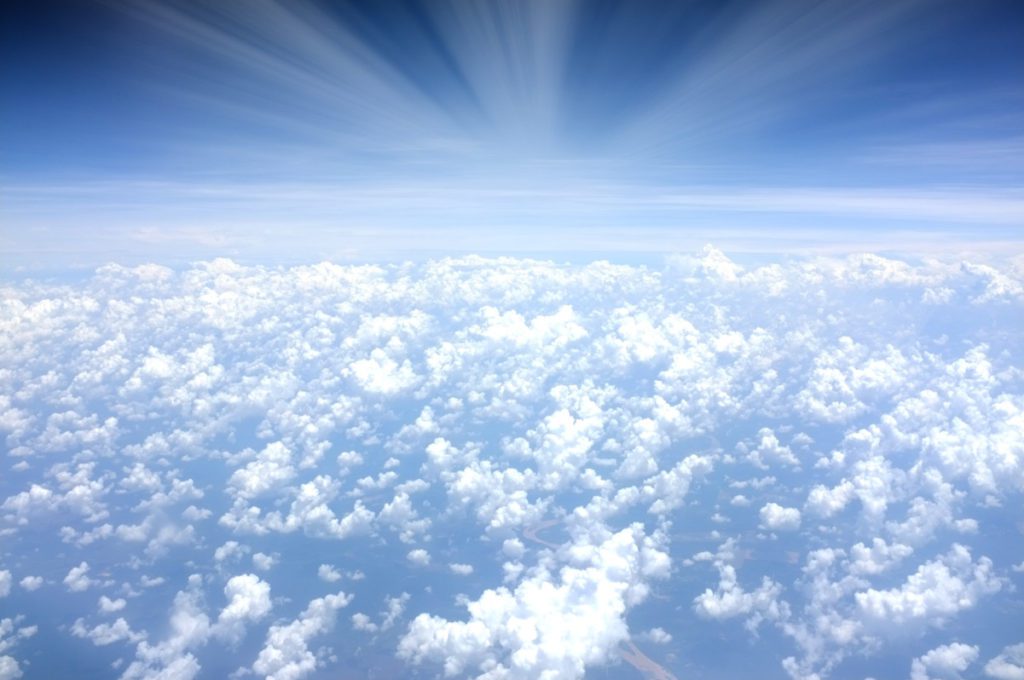
As any actuary who’s been in modeling for any time knows, there are multiple trade-offs in creating your model. We’ll be presenting some of those tradeoffs over a series of articles. This is the first, discussing the inevitable compromise between model detail and run efficiency.
AN UNLIKELY CASE STUDY
There once was an insurance company with a block of Universal Life insurance policies. Let’s call them Insurance General Company (IGC). Like all insurance companies, IGC regularly performed a valuation of the block, whether for reserving, cash flow testing, or perhaps cost of insurance (COI) rate reviews. And, like all insurance companies, IGC had a setup that required simplifications of many model factors. They grouped policies by similar issue ages, issue dates, underwriting classes, average sizes, and policy features. They looked at policy values on a monthly time scale, and they grouped some of their underlying assets as well. They had to do this in order to get their models to run in a reasonable (i.e. useable) time frame each period.
Now, to be fair, the following example is an anecdote (or a composite, if you will), not witnessed first-hand by either of us. But we’ve all seen similar situations too many times with too many models.

However, because of all the simplifications, when the next valuation period came around (the next month’s policyholder reserve calculation, for example), there would be differences between the forecast and the actual values. Perhaps the investment credits didn’t perfectly line up between the group and the individual policies. Perhaps the weighted average of the COI charges diverged throughout the month differently in the model compared to actual. Regardless of the specific reason, the future values would differ from the prediction. And there were questions about why. Might this be a modeling problem? Could a better model reduce these variances and provide better results in the future?
AN INSIDE-THE-BOX SOLUTION?
In order to test that question, IGC proposed to eliminate modeling simplifications and create a daily projection of every single value for every single policy on their books. No more monthly time steps, no more policy groupings, no more “average age” rather than exact age. No more variance?
Their actuaries went to work. They built a model point for every policy. They ensured every single possible COI table existed. They made sure that all interest credits and fees were projected on the exact day of the year, every time. They even went so far as to ensure they had programmed in the exact number of days of each month. Once they had tested a few policies to make sure they knew it would work, they hit [RUN] and waited for their perfect results.
And waited.
And waited.
In the end, it took 3 days of computation time to run one day’s worth of policy values. If this were a 20-year forward look (like in cash flow testing), it would be almost the next century before they’d know the answer. That’s just not feasible.
The added computation and data storage requirements necessary to calculate all of the daily policy values created such a burden on their processors and their data management system that the model became unusable. It didn’t actually answer the question it was asked, because it couldn’t.
Now, for a small block of very simple policies, this might be reasonable. But for IGC, and for virtually any other insurance company, nothing profitable is ever small or simple. Real insurance is complex, with a lot of moving parts, and (if you’re doing it right) you’re selling a lot of them, so you quickly overwhelm some models that can be useful when trying to apply such precision.
HARDWARE LIMITS FORCE UNSATISFYING COMPROMISES
The trade-off between detail and run efficiency has traditionally favored model efficiency. If you thought you might have a problem with tomorrow’s values, but couldn’t get an answer about tomorrow for 3 days, then by the time you do get the answer your opportunity to solve the problem has passed.
This is a familiar challenge for actuaries. They have to simplify their models, or else they’d never get anything done. This is due to the limitations from in-house (on-premises) fixed capacity servers, processors, and data transfer tools.
Because of these limits, actuaries have long resorted to simplifications like grouping model points, selecting longer time steps (months, quarters, or years), and creating average or weighted-average assumptions. These lead to model error and drift over time as the initial assumptions don’t quite match future experience.
And not every single simplification is bad. Monthly time steps are probably perfectly fine for almost every actuarial calculation. But in general, actuaries don’t really like the artificial limitations imposed on them by hardware and software. So is there a better way? Other than waiting until 2079 for this year’s cash flow testing results, that is.
BRUTE FORCE SCALING IS INFERIOR TO THE CLOUD
The classic way to solve this is to just brute force an answer. Buy more servers! Upgrade the processors! Expand the data warehouse! All hands on deck!
Ultimately, though, that is a costly and wasteful exercise. First of all, you’re not likely to need your maximum processing requirement (such as during valuation periods) for the majority of the year. If you scale to always be ready to meet that need, you’ll have unused (and thus wasted) capacity the rest of the time. Plus, your data storage requirements aren’t static. Each valuation period you’re going to add another layer of results, and that either means a repeated process of storage acquisition or the need to expand on-demand.
A better way to create processing flexibility and on-demand storage capacity is with a cloud-based system. Using cloud servers, both capacity and storage are flexible and scalable to the user’s demands, in real-time. There is no excess capacity that goes unused, and there is no repetitive hassle of one-off increases to storage space.

The advantages of cloud computing are legion, but those relevant to this discussion are quite clear: with cloud computing, there is no model computation limitation. Flexible access to processors could have taken that 3-day model of IGC to 1 day (by tripling the # of cores used), ½ day (6x), or even just a couple of hours (50x). You can’t do that with standard fixed processors.
Plus, if done correctly all results can be automatically stored, and always accessible, within the cloud, which means no more back-and-forth with the procurement department on how much you really need. Saving you time and money. But, more importantly, getting actuaries back to what they do best – analyzing complex problems – rather than wasting time dealing with infrastructure issues.
Admittedly, not all models will be able to run on cloud servers or with cloud storage. And even those that do will probably still have some simplifications, in order to keep the volume of results for the actuary to review at a manageable level. Yet cloud computing offers a great alternative to historical limits on model information and usability from the efficiency trade-off.
And as insurance products get more complex, cloud computing and storage is the clear choice to support the future of actuarial work.
At Slope, we’re experts in applying cloud technologies to actuarial models. If you’d like to learn more about how leveraging the cloud can improve actuarial work, give us a call at 855.756.7373.