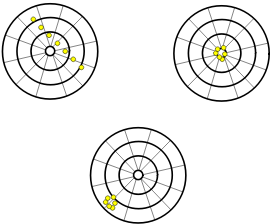
We promised a series of articles discussing actuarial model trade-offs, and dang it, we’re going to keep our word. Our first trade-off article was about Modeling Efficiency versus Detail, and you can read that one here.
Today we’re discussing another trade-off that many actuaries contend with on a daily basis: how precise do you need to be? How precise can you be? How precise should you be?
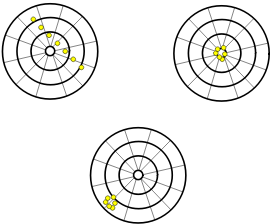
This is not the same as efficiency vs. detail. You can have an efficient model with insufficient precision, and you can have a slow model with not enough accuracy. They may seem similar, but they’re not. They are related, though.
Model efficiency is more about the runtime. You answer the precision / accuracy tradeoff by considering these two questions:
Precision: What are the error bounds of my answer?
Accuracy: Do the error bounds contain the true value?
The precision vs. accuracy tradeoff can show up in your assumptions or your output sets. Suppose you’re running a Cash Flow Testing model with stochastically-generated future paths of Treasury rates. You do this because you want to know how likely it is your assets can support your liabilities across the horizon.
The precision of your results will be how tightly-clustered your final surplus values are. If the median scenario has an end result of, say, $53 Million (M) in Surplus, with a range from -$25 M to +$160 Million, that’s not very precise. Not as precise as a model that has the same median value of $53 M, but only ranges from $37 M to $83 M. [Whether you judge based on mean, median, mode, or some other metric altogether is a completely separate discussion.]
The accuracy of your results is the likelihood that your end result actually contains the future that is to come. Let’s say, again, that you run real life forward for twenty years, and the final surplus would turn out to be $29 Million. This would demonstrate that the first model was not precise, but it was accurate (actual turned out to be within the error bounds). The second model was much more precise, but it was not accurate.
Physics has a pretty good handle on what it means to add more precision versus adding more accuracy. You can read all about how to determine how precise your measurements are by browsing through that textbook chapter. We highly recommend it.
So, which is better, precision or accuracy?

Actuaries obviously want both.
But the truth is, it’s quite difficult to get both. Insurance models simply have too many variables, with too many options for each, and run for too long a time frame, to realistically get both low variability (precision) and high accuracy.
That’s because insurance is a complicated, long-term contract. Especially life insurance, which may last for 80 years or more. Can you accurately predict 80 years of mortality improvement? Well, sure, if you widen the error bounds and reduce the precision.
Why Do We Want Precision, Anyway?
We seek precision from our models because in between now and the future we’re planning for we will absolutely need to make decisions. If we can make some of them now to ward off potential undesirable futures later (that are predicted by the model), why wouldn’t we? If we could make those later decisions with better information (again, because of predictions from the model), and reduce the chance of ruin, why wouldn’t we?
That’s why you’re building a model, anyway. So it can be useful. You’re not just building it for kicks and giggles.
Which means that to balance both more precision and more accuracy requires a skilled professional like yourself.

How to Perform That Balancing Act – 3 Tips
To give you some additional support as you perform this tradeoff between precision and accuracy, we’re including 3 tips to develop your skill.
First, measure the sensitivity of your results to the various assumptions included in your model. Remember, some elements are not assumptions and some are. Mortality rates are an assumption. Commission rates are not. Whether agents choose heaped or distributed commissions, however, is an assumption. Test the various assumptions within your model to see how sensitive your results are.
Prioritize your refinement according to the assumptions which will give you the most change in precision – i.e. go for the ones that add the most variability to your results first. You may find yourself wishing that your actuarial software was easily set up for this kind of sensitivity testing. That’s good! That mean’s you’re expanding the frontier of your model capabilities. What’s not so good is the absolute nightmare some software systems make this kind of thing. Wouldn’t it be cool if a software just did what you told it to do, every time?
Then again, you’re smart people. You can figure it out. Might take a few extra spreadsheets and databases to get there, but we have the utmost confidence in you.
Second, practice getting comfortable with less-precise numbers. The actuarial world seems to be addicted to more decimal points wherever possible. Try backing off to 2 significant figures for most of your communications. Rather than saying, “There was 31,347,236 dollars of premium last month,” try reporting that there was “31 million of premium.” That extra precision, of considering dollars when you’re also considering millions, doesn’t really impact the accuracy. (Because let’s say you were off by 500 or even 10,000 dollars. Would that be a material difference compared to 31 million? No.)
The more comfortable you are with understanding what values are good to be precise about, and which are good to consider in much more general terms, the better you’ll be able to communicate your results to the rest of your team. Because you’ll be focused on more accurate values, not just more precise ones.
Finally, aim to provide more variance in your conversations with peers and even non-actuaries. What if, when you are asked for an estimate of the income from a line of business next year, you provide more than a best-estimate? What if you provide an error bound, too? “We’re looking at 750,000 dollars, plus or minus 100k.” You’re much more likely to be right with that than just saying “750,000 dollars”. If income next year turns out to be $825k, are you really wrong? Are you any more wrong than if it comes in at $675k?
Both are 10% off of the original estimate. And both would be equally “wrong” if you had provided only that point estimate. Both would be “right” if you had provided an error bound (and some kind of confidence interval) along with the best estimate. The second way, which includes adding a confidence interval, allows you to enhance your professional credibility and gives you something to measurably refine over time. As you become more “precise” and more “accurate”, your predictions will be given more weight. Allowing you to be more influential in your company’s decision-making process.
Conclusion
In order to provide more actionable model outputs, you need to find ways to increase the accuracy of your results. This may also include getting more precise about some model components. Early on, you’re likely to introduce a bit of culture shock as you introduce sensitivities and error bounds around your assumptions and your results. That’s good. Go with that. It means you’re getting better.
Remember – muscles don’t grow stronger without strain. Exercise yours in the precision vs. accuracy tradeoff and see better results in the future.