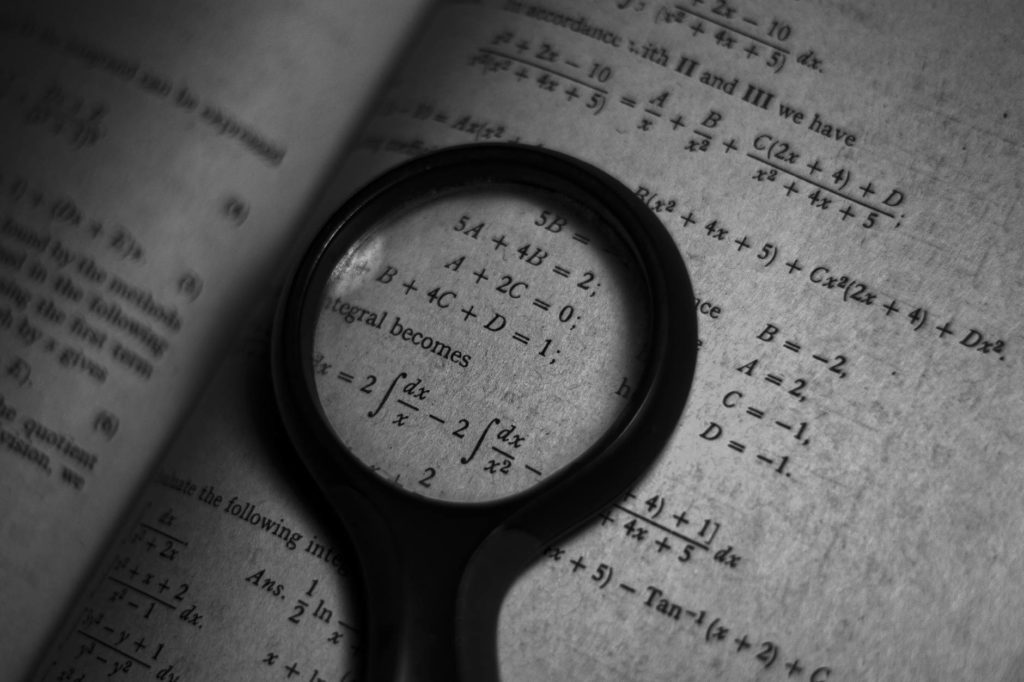
Upgrading your actuarial functionality ultimately involves change. A necessary part of that is converting your models to use modern actuarial software.
We’ve been supporting your efforts at such conversion process with our blog series, the 7 Steps to converting actuarial models, which continues below. First, a quick recap:
- Step 1 – Determine you need a new modeling system (part 1) (part 2)
- Step 2 – Build a case for conversion.
- Step 3 – Compare candidates (understand system differences)
- Step 4 – Make sure you’re not making a bad decision.
What’s next? Step 5 – getting to the actual work.
1. Inventory current models; prioritize conversion efforts
The first thing you’ll need to do is to create an inventory of all your models.
To be very technical:
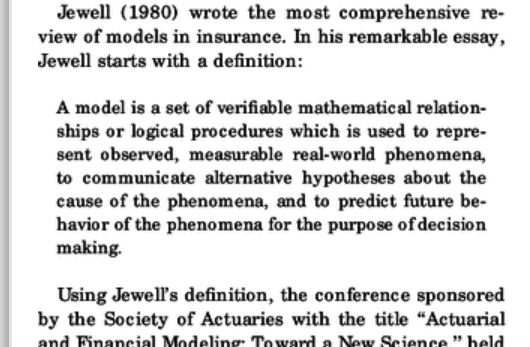
We consider this a little more specifically. For actuarial modeling practice, we define a “model” to be a combination of insurance products for a financial process.
Actuarial model: a combination of insurance products for a financial process.
That is, you may have many different models in practice. You might have both a Whole Life – Pricing model and a Whole Life – Valuation model. As well, you might have a Traditional Life – Forecasting model. In this case, Traditional life might encompass both Term and Whole life liabilities and the asset portfolio supporting those combined liabilities.
These may all use the same or different Whole Life “products” (pre-defined sets of calculations), and they will definitely have different steps involved.
Your pricing model may not need an extract of in-force policies, but your valuation model certainly will. Your pricing model may not need economic scenarios, but your forecast model most definitely will.
In addition to the liabilities, your forecasting model may also incorporate asset cash flows as well. You may choose to run with your portfolio of invested asset products or with pre-determined investment assumptions.
The point is, you have a lot of options for which models you will need to convert to your new system.
And remember, all of your models may not already be in your current system(s?). You may still have some which are housed in Excel files or run through R programs, for example. These should be in your inventory as well.
Plus, you’ll also want to think about not just the current state, but what do you want the future state to look like? Just because you now have 30-something models in your system and 5 separate Excel spreadsheets, is that what you want to have going forward?
Model conversion is a great time to reconsider just what it is you’re trying to do with all those models. A comprehensive view of what they are, and what you want them to be, is essential to making good decisions at later steps in the process.
[If you’re still looking for ways to convince others to make the investment in converting your models to a modern actuarial system, check out our invitation-only white paper: “12 Hidden Benefits of Model Conversions”. Get it by sending an email to info@slopesoftware.com and requesting a copy.]
Once you have your inventory of models, it’s time to start planning.
2. Set deviation tolerances
Your deviation tolerances are how much difference you are willing to accept in the cash flows or balance sheet items that are outputs of your two systems.
There can be many different reasons why one model produces a value different from another. Cash flow timing (end-of-month vs. middle-of-month), order of operations (expenses before COI charges or after?), or even rounding rules can create differences.
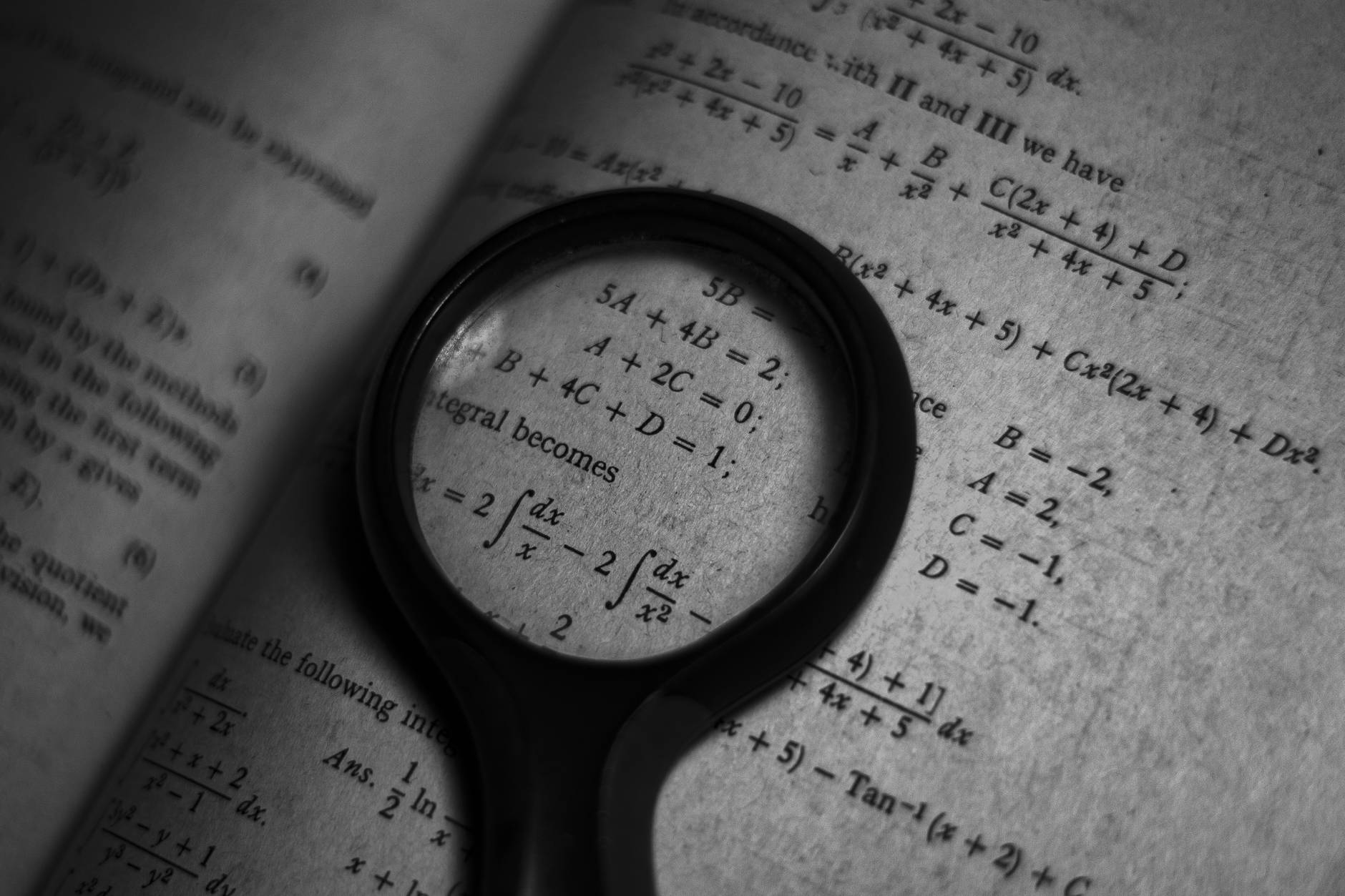
Maybe not big differences, but differences.
And if you’re trying to exactly match cash flows, differences stand out and draw you into investigating them.
Which (see step 6 below) can become a drag on your project plan. If you can avoid that by setting appropriate tolerances at the beginning, you can avoid unnecessary delays of the project.
This is a task that some conversion efforts don’t consider up front. When they get into the nuts and bolts, however, it comes back to haunt them.
We suggest starting with fully independent variables, and then those that are immediately connected to those independent variables, and then expand out from there.
By this we mean, it doesn’t do much good to compare net cash flows (very dependent values), if the underlying elements (independent values) aren’t matching very well in the first place. Sure, 5 + 5 = 10, but so does 3 + 7 and -5 + 15. If you’re way off on the base elements, how can you trust the aggregates?
Setting too low a tolerance for difference is going to delay your progress. You’re going to ultimately end up chasing down immaterial elements, like rounding rules. And setting too high a tolerance for difference is going to add some distrust to the models, because your new model results are just too far away from the current sensibility to be accepted.
For example, let’s take that 5 + 5 = 10 equation from above. If you set a 1% tolerance, and got to 4.94 + 5.07 = 10.01, you would pass according to the aggregate value (10.01 is less than 1% different from 10.0) but fail at matching underlying elements (4.94 is 1.2% lower than 5, and 5.07 is 1.4% higher than 5).
Which would indicate a need to dig deeper into the model and understand why your values aren’t within your stated limits.
A reasonable progression for validations might look like this:
Number of policies in-force at specific points in time
This is influenced by your assumptions about mortality, lapse, contract termination, guarantee periods, etc. This is a good place to start because it’s fairly independent of other cash flows. If you can reproduce some basic actuarial mathematics between the two systems, then you can be confident there aren’t fundamental deviations that will create downstream differences.
Policy cash flows
This is elements like premiums, commissions, expenses, dividends paid, reinsurance, etc. These are amounts that are tied to independent elements like policies in-force. As a result, these can be very different at the model level, if the underlying values aren’t fairly closely matched.
Aggregate balances
Once you’ve got policy values, premiums, investment income, and other cash flows tied correctly you can start looking at reserves, surplus, capital, and so on.
Now, the bigger issue is probably not just the values. It’s what do you do with different values?
Or, in other words,
What is close enough?
How far apart can your old and new models be before you’re comfortable to move on to the next level?
In the last two segments, policy cash flows and aggregate balances, it may also be helpful to consider lots of ratios, rather than actual values. Because if your in-force policies differ by, say, 2% at the end of your projection (and you’re okay with that), then shouldn’t your reserves and benefits paid be differing by about the same amount? What won’t differ (much) is the ratio of premium to amount of insurance in-force, for example, or the reserve per unit.
It’s fair to ask, though, so what? So there’s a difference between the two. Is it material? Is it substantial?
The point is, you want to determine a tolerance, or an amount that you’re comfortable allowing values to deviate before you start questioning all that’s been done.
An example might be, “We want the average ending surplus of our Cash Flow Testing models to be within 5% of the prior model.” Or, “We want a pricing IRR that is no more than 10 basis points different from the prior model.”
Sure, all the valuation actuaries are going to want “same reserves, to the penny”. And the pricing actuaries are going to want “IRR is the same, to the 0.01%”. But are those reasonable?
Even more, would a difference in one of those values significantly impact the decisions you make about that business?
If so, how big of a deviation would be enough for you to make a different risk management decision?
Frankly, not having this discussion and prioritization before model conversion is often where the problem starts. The differences between values that arise from comparing across two fundamentally different systems is where we see many model conversion efforts start to go off the rails.
We get it. It’s exciting to have a new modeling system to play around in, to learn about, to get some fancy new report out of.
But when you just jump in with building models, without any kind of thought about what’s a good basis for comparison, you’re setting your team up for an inefficient conversion process.
What’s the risk?
When differences arise (as they are virtually guaranteed), conversion actuaries get stuck chasing immaterial inequalities that don’t ultimately matter for the model management or decision-making going forward.
The majority of the time, this isn’t because of the new system. Most often, you’re struggling to understand what was in the old model system (that you’re going away from anyway!). This feels like a futile effort.
Instead, you should probably be spending a lot more of your time validating that the new system has what you want it to have.
Unfortunately, without some understanding of what is an acceptable difference between the two at the start, it can feel like you never get to the end.
The way to circumvent that is to set your variance tolerances at the start and understand that there are going to be some differences. As long as your agreed-upon tolerances are met, you can remember that, in the end, it’s a simplification that may be useful, and can move forward.
So – once you’ve identified some tolerances for various elements, you’re ready to start converting.
3. Convert one model first as a test run
Conversion may seem intimidating. You’re comparing everything from your admin download to your processes for wrangling data to your actuarial mathematics. There’s a lot going on.
You’re going to learn a lot that you didn’t know.
You may find errors in your prior model. We saw this happen with a client. There was an error in their spreadsheet, which they didn’t know about until they were investigating why their model values didn’t match the spreadsheet. It’s okay, they’ve fixed the problem.
[Incidentally, that’s another of those Hidden Benefits of Model Conversion – #7 – Discover Latent Model Risks. Get it here.]
All of that may seem like an effort that expands beyond what you thought it would be when you started.

Image credit: TCD/PROD.DB / ALAMY via Telegraph.co.uk
The point is, you don’t want to get too ambitious and over-sell the process. You want to ensure that you have adequate time to thoroughly understand your new models and why they differ from your old ones.
There’s some psychology at work here. Anchoring is the idea that people are more comfortable with the things they know than something new and unfamiliar.
Actuaries (and their auditors, and the finance professionals they deal with regularly) are going to be more comfortable with the old system. Totally understandable. But the point is, it’s the responsibility of the conversion professionals to guide them along the path to the new way of thinking about models and modeling. In this situation, you don’t start by going from 0 to 100 instantly.
That’s overwhelming and will initiate barriers to adoption that you don’t want to have to break down again.
Instead, you’ll need to ramp up. You’ll need to scale up your production process so that everyone can be comfortable with the fact that you’re going to be introducing something new and that you’re going to be producing different results (not necessarily “good” or “bad”, just different).
Which means you should pick one model (one set of products for one process) and convert that first.
There are a few different perspectives of which model to select first, along a spectrum. At one end would be “simple” models and at the other are “complex” models. Here are some examples:
Simple | Moderate | Complex | |
Products | Term Life | Fixed Deferred Annuity | Annuity Portfolio |
Process | Pricing | Cash Flow Testing | Investment Strategy |
Simplicity/ Complexity | fixed interest, no dynamic policy interactions | introduces assets and scenarios | multiple risk profiles, dynamism in both asset and liability management |
Those who advocate starting on the “simple” side say, “We need to learn the system first before we can worry about conversions. We don’t need to get into all the details right away. We’ll be able to add that later once we know what we’re doing.”
Those on the “complex” end say, “We need to know if the system can handle anything we throw at it. We don’t want to get halfway through and then find out it’s insufficient.”
If you pick a model in the middle, you’re splitting the difference. It’s probably likely that the majority would choose something like this, rather than the two extremes. From here, you’d have a good view of what it might take to add the extra complexity of your more robust models, and how fast you could build out the simple ones.
All three perspectives are valid, and would be appropriate for various lines of business, risk appetites, and transition timelines.
Ultimately, it’s up to you to pick one of the models on that spectrum and move forward. Because you already have an inventory (step 1) and a set of tolerances (step 2), this is actually going to be easier than you thought.
You’ll convert the model, you’ll document your process, and you’ll present your findings. Then, you’ll move on to the next step.
4. Complete an after-action review
Once you’ve gotten a model “converted”, you’re going to want to bring in others on your team to help evaluate the conversion process.
You’ll want them to look at the way you have set up the model, the processes, the data flows, and any modifications you’ve made to anything that comes standard from your vendor.
You’ll want them to evaluate not just your comparison of values between the two models, but whether you have created as simple a model as necessary. You might have taken shortcuts that will ultimately result in additional work-arounds later. You might have added in features that aren’t appropriate, just because you could. Is your documentation (not only of the model, but of the conversion) sufficient?
[See virtually any ASOP, which will have a section on Documentation. For example, ASOP 41 – Communications or ASOP 56 – Modeling.]
Now, this is admittedly not so fun and sexy a step. It will feel like it’s not productive work. And it may result in some criticism of the way you’ve put together your model and some of the decisions you made along the way.
But it is incredibly valuable.
This kind of review will inform the way you go forward and ensure that any less-than-ideal decisions you have made in the first conversion will not be repeated in later efforts.
Thus increasing the chance that your conversions become a source of pride and actually enhance your future work product, instead of porting one bad process over to a different structure (system).
5. Set up structures and repeatable processes
Based on your first model conversion and after-action review, you now have a feel for what it will take to convert your other models.
The important thing is that you’ll be making decisions based on data, not on conjecture.
At the outset of your conversion process, you just didn’t know. You didn’t know what you might find. You didn’t know the intricacies of the new system. You were bound to discover some of Secretary Rumsfeld’s famous “unknown unknowns”.
Once you uncover them, though, you’ll have a greater understanding of what you’re working with and what it takes to solve those problems.
Also, you can set up repeatable processes that you will follow in the future. Will you consider in-force file sources first, or data outputs? Will you prioritize policy values or reserve balances?
How often will you perform peer review? What does it look like if the peer finds something wrong? How is the “request for refinement” process? Is the documentation of model differences sufficient? What about signing off the new model and putting it into production, who does that and when?
Once you have finished the first model, you can now address some of these secondary issues. You’ll want to ensure that they don’t slow down the process of actually building your new models and comparing them with the old ones. At the same time, you also don’t want to leave all of these for the end. Waiting too long could result in you forgetting some or much of what you did in the early stages.
And it could unnecessarily delay the adoption of the final models themselves, when your auditors are looking for documentation of comparisons, or formulas, or assumption tables, etc.
If you set up appropriate processes at the outset, everything goes more smoothly.
6. Plan timing of work
Okay, you’ve got a feel for how it will work to convert your models and all the ancillary steps that will be required. Now it’s time to apply that feel to your inventory (from step 1).
You can estimate the degree of complexity of the model, the number of steps in the data pipeline, and the after-model needs (reporting and storage) to get a baseline for comparison.
Then you can apply that baseline, scaled up or down as appropriate, to your remaining models.
Don’t forget that you’re not likely to have 100% of your time devoted to model conversion.
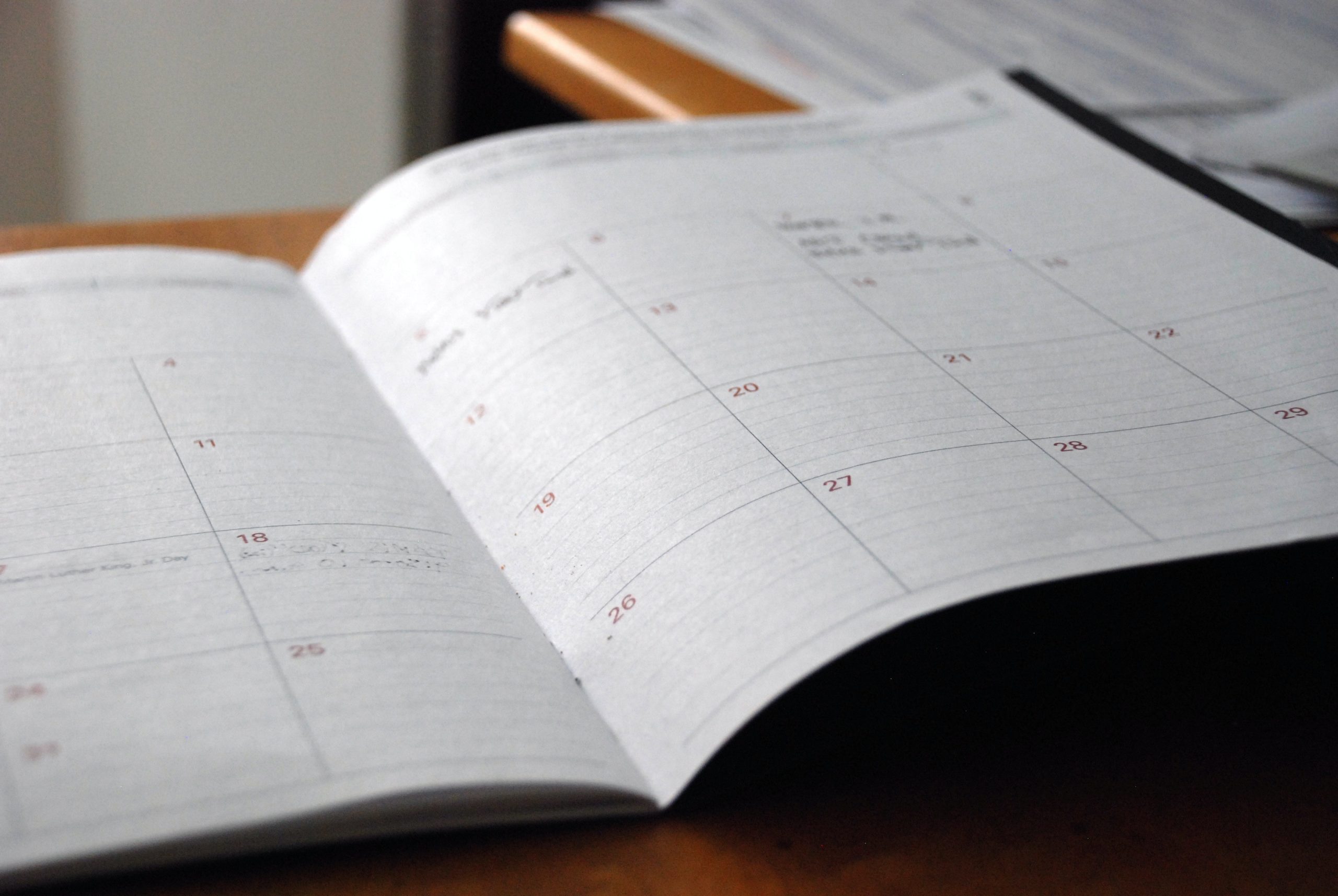
You may need to work around month-end, quarter-end, or year-end reporting requirements. You may also need to complete regular experience studies or benchmarking, depending on your responsibilities. You may need to also plan some flexible time in case your conversions don’t go as smoothly as you hoped.
This is probably the biggest unknown of the whole thing. We’ve seen efforts that stretch from a planned 18 months into 4 years or more.
Not here, of course. SLOPE users see incredible speed-to-value. “Within 24 hours I had a working model I could actually get results out of.”
Dorothy Andrews, The Actuarial and Analytics Consortium
However, that is mostly because of how little was understood of the prior model. Usually, the new model is clean and clear. You’ve got good documentation and everyone involved knows exactly what was done and when.
With your historical systems, there are potentially decades worth of older decisions that either weren’t documented, or were but the documentation was lost, moved, or archived.
Plus, there could have been plug-ins or work-arounds affecting calculations that just aren’t as visible [rhymes with Schmalkuflex, anyone?] or readily understood. Maybe not even working correctly.
That means while you think you know what’s in those older models, you probably don’t know. So your conversion efforts end up stretching out longer than you intended, because you keep finding more unknown unknowns.
That’s why you’ve got to plan for some contingency in your conversion timeline.
Again, discovering unknown unknowns is usually not the case when you’re in production in a modern actuarial system. Those systems are often designed for much more transparency and flexibility than historical systems. We can’t stress that enough. The discovery comes at the point of digging in to old, undocumented, potentially black-box-type modeling systems.
Once you’ve got your plan set, you’ll be ready to move forward.
7. Regularly refine the plan as you progress
For smaller blocks or companies with fewer product lines, conversion can be a simple and straightforward exercise. You’ve completed your pilot conversion, you’ve applied your learnings to what remains, and you move forward.
For larger blocks, or more complex models, you may continue to discover unknown features of the models or processes as you go along. This may inspire you to adjust your timing of the plans.
A good cadence would be every month or so, or after every major model is completed. If your timeline is shortening you can brag about delivering sooner than expected. This could happen (and probably should) if you become more efficient with your model building as you learn your new system.
If it’s extending, because you’re seeing just how much you didn’t know about your models before, take the opportunity to educate your audience. You will need to be clear and set appropriate expectations for final delivery of converted models, obviously.
At the same time, you have the chance to illustrate to them just how necessary the model conversion effort was.
[aside – you could also request the “12 Hidden Benefits of Model Conversion” – send an email to info@slopesoftware.com to request it]
This is the Actuarial Control Cycle in practice: make a plan, execute, refine, re-execute.
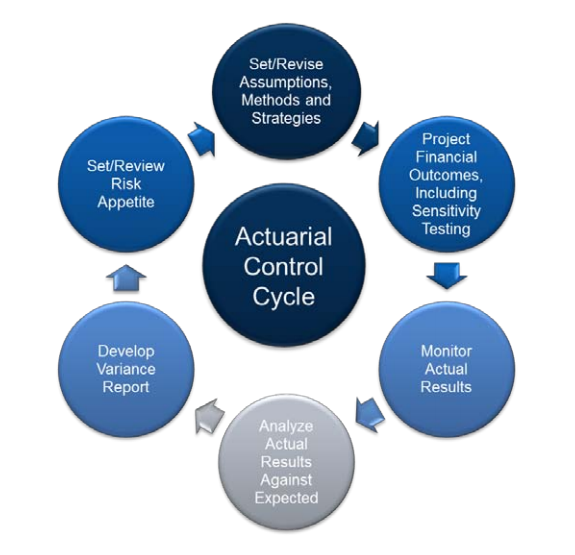
Ultimately, you’ll keep refining and refining until it’s all done!
And, frankly, shouldn’t this be the way we do all of our business? Set a plan, execute, learn, refine, set a new plan, and repeat.
Conclusion
Okay, here’s the TL/DR version of the practical steps to converting models to a modern actuarial system:
- Inventory and prioritize – if you don’t know what you have to do, how can you make an informed decision on what to do first?
- Set deviation tolerances – without this, you’re likely to get distracted by immaterial differences.
- Convert one model first – to get used to the process.
- After-action review – so that you can get some independent evaluation.
- Set up repeatable structures and processes – to create some appropriate benchmarks and consistency.
- Set a timeline and get started – don’t forget about your “regular” functions too.
- Regularly refine the plan going forward – as you do in everything else.
It may feel like a lot, but it’s worth it. Soon you’ll have all your old models in your new system and you’ll be more productive than you ever thought you could be.