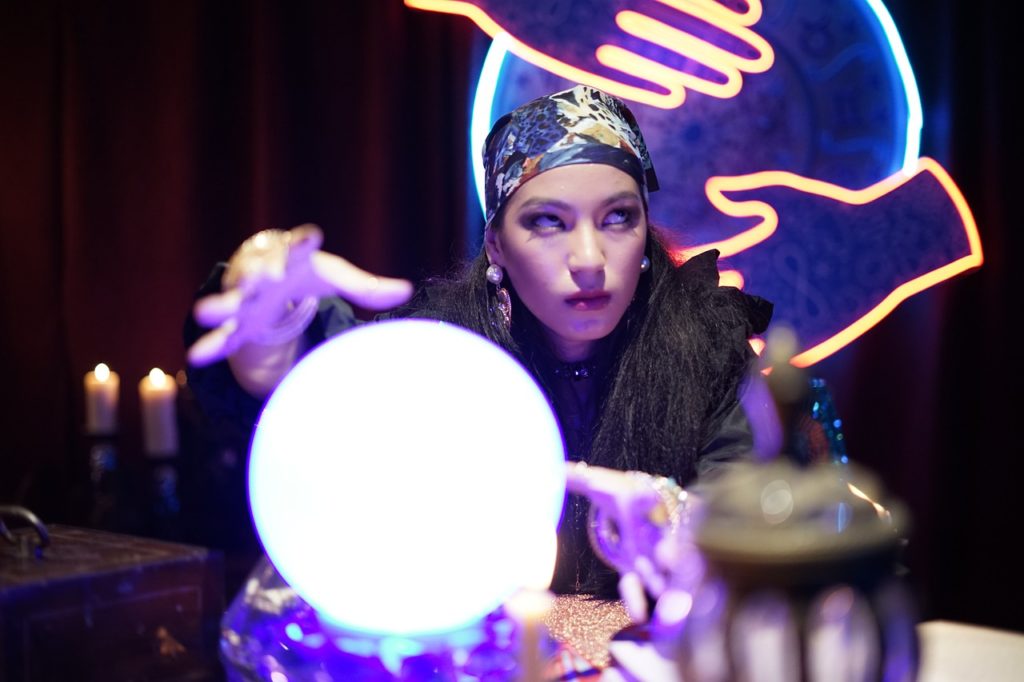
You might not believe it, but it’s true: actuaries have the ability to see the future.
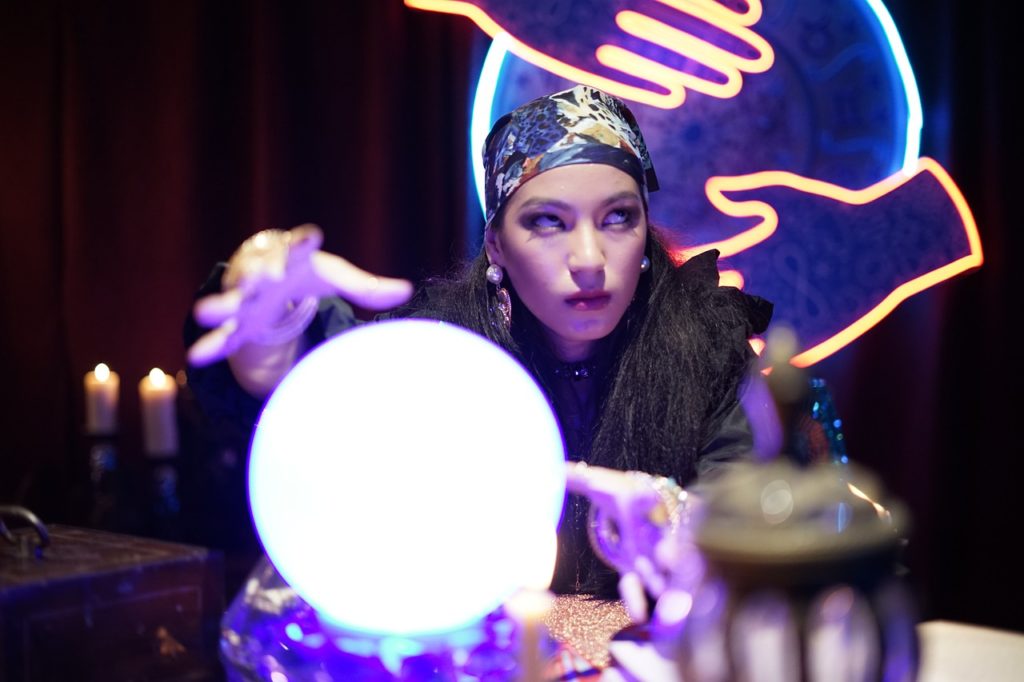
And not in the exotic, sci-fi movie kind of way. We’re not talking Minority Report.
Nor do we just mean the standard “Next year’s loss ratio is predicted to be 62%, plus or minus 3%” type of prediction.
That’s a forecast, and it has its place. The type of seeing the future we’re talking about in this article is of the What if you could get the expense report three weeks sooner? kind.
The idea here is that when actuaries employ scalable technology (like that found in cloud-based reporting and forecasting systems), they can get access to information now that they used to wait days and weeks for. It’s like bringing future results of three weeks from now into the present. Which is a kind of seeing the future, if you think about it.
Let’s dive into this with an example.
The setup – it’s okay for now
The typical situation inside an actuarial department goes something like this. The actuaries need a whole lot of computing power to run their quarter-end processes or year-end analysis. Especially since the introduction of Principles-Based Reserves, and with GAAP LTDI and IFRS-17 coming online soon, this is becoming more and more relevant.
Let’s say they have 100 scenarios they like to run (maybe 10 different economic scenarios, for one base case and 9 sensitivity cases or attributions from period to period). Each scenario takes 2 hours to run. So we’re up to 200 hours of run-time each quarter-end cycle. All this has to get done by the start of business day 4 (BD4), so you have 200 hours to run in 3 days. Let’s say it takes a whole day to prep the model and test to make sure all the plan codes exist, errors aren’t going to show up during runs, etc. Leaving you 2 days of calendar time to let the system run.
So you’re down to 200 hours of run in 2 days, or 100 hours / day.
Simplistically, you need something like 4 processors (cores, in IT-speak) running 24 hours a day to get you those 100 hours of processing. It’s a happy coincidence that your resource planning team was able to get you those 4 cores in a machine solely dedicated to the actuarial team, so nobody else can interfere with the process.
Our actuary doesn’t want to risk impacting those who are downstream, so her checklist includes triple-checking everything before initiating any full projection runs. Sure, it takes coming in a few hours early for a few days, but it’s worth it to reduce the risk of missing the reporting deadline.
So, bright and early at 6 am on BD2, the actuary kicks off the runs, set to churn while the actuary hopes everything was done right. The whole setup runs through early morning on BD4, and then the actuary can check reports and results.
And at this point she hopes everything’s right, because she doesn’t have time to re-run. Remember, the company could only afford enough capacity to just barely meet peak demand. Otherwise, she would have bought twice as much, gotten everything done in half the time, and been able to re-run something if an error popped up somewhere.
This time, everything worked well. No errors from missing data tables; no obscure, inexplicable values requiring investigation; no machine failures.
The actuary compiled her reports throughout the bulk of BD4, created the graphics and analysis, and sent it off to the finance team at 3:30 pm, just in time to meet their downstream deadlines.
Phew! That was close.
What’s next?
This is all well and good, for the need as it stands now. However, what happens if the company needs to start running more sensitivity tests or attributions? Or the block of business grows significantly over the coming year, doubling in size and now you need to run twice as many model points? Or what if the economy suddenly enters a wild phase, where 10 economic scenarios is not enough and there’s a request to run 100? How should an actuary get ready for some of those situations where need expands to 2x, 3x, or 10x of what it was before?
The brute force way would be to just double, triple, or 10x capacity. Buy more machines. Spend more IT time (and budget) getting them set up.
And, have more unused overhead during non-peak times.
Is that the best way to go? It used to be the only way, when scalable technology didn’t exist. Now? There are other options. Why might the brute force way be inefficient? It’s pretty clear to see what needs to be done. The problem is that the need is nowhere near constant, and nowhere near the peak.
What a waste
Let’s take a look at off-peak times, when you’re not maxing out your processor capacity for days at a time.
In between high-demand cycles the actuaries don’t need a lot of parallel processing all at the same time. Let’s say their baseline is more like 1 hour of run-time each day.
So, how do you plan for this? Do you give the actuaries a bank of servers and processors that can cover the peak need at the moment (100 hours per day) and then sits around unused during the rest of the time (99 hours per day)?
Or do you make them create work-arounds and simplifications (model point groupings, selective sensitivities, shorter time frames, etc.) to get those results completed using limited resources, i.e. “whatever you could afford”?

Neither way is an efficient solution. Either there’s a waste of resources and capacity, or there’s shortcuts to the process which introduce additional model risks and can result in lowered model effectiveness.
Technology to the rescue
Enter “scalable infrastructure”. It’s a new world, now that cloud computing is readily accessible. Using demand-matching technologies, cloud service providers can expand the capacity of what’s available to end users as demand increases, matching the needs dynamically to reduce unused capacity.
[Technically, from an IT perspective, this is “elastic” capability, because scalability applies to how the capacity grows with user base and storage needs, elastic goes with balancing instant load demands.]
This is made available often through public cloud technologies. There are “cloud tech providers” who have a bank of calculators available for you to use, whenever you need it. The top names are familiar ones: Amazon, Microsoft, Oracle, Google, and so on.
Those providers offer services to many different industries, not just actuaries or insurance companies. Since those other industries don’t have the same kinds of demands for computing power that the actuarial world does. Which means their peaks happen at different times. Service providers aggregate all of those smaller needs from hundreds of different companies into one big mixed bag of requests. It’s like diversification in your investment portfolio, only in access to computing resources.
As a result, cloud service providers can make X (some ridiculously big number) of units of computing power available to their clients at a fraction of the cost that any one of those clients could have bought [X] for themselves. As a result, the wasted capacity factor diminishes considerably.
This gives users of elastic cloud-based services access to the computing power they need, without forcing them to buy up their maximum capacity all at once and let it sit around, unused.
Think about that service provider. Suppose they have access to 1,000,000 cores for calculation, which can be allocated across their 500 different clients as demand requires. [Big round numbers for ease of comparisons.] One of those clients happens to be our actuary friend from before.
The provider makes constantly available 2 cores, so that she can always run whatever she needs during off-peak times. And when she has a larger need for computing power, sat at the beginning of the quarter, they can allocate something like 100, or 10,000 of their 1,000,000 to that actuary without missing a beat.
She gets access to capacity when she needs it, the company doesn’t have to shell out for unused capacity when she doesn’t, and the service provider gets to diversify their resource use.
Seems great, right? A very relevant question is, What happens when you want to expand?
Scalability allows for expansion
Let’s investigate, then, how our actuary would benefit from the opportunity to increase capacity using scalable technology.
Again, let’s revisit the example from before. If the directive comes down to analyze 100 economic scenarios instead of just 10, for each of the baseline and 9 sensitivity cases, now your computing requirement just went up by a factor of 10. Under the fixed capacity setup, this is most definitely not going to be finished before BD4. Meaning your report is going to be late. Maybe by weeks.
Even worse, there’s no way to make it better, because that’s as fast as the setup can go.
But under the scalable setup, capacity increases as demand does, with very little change in time to results. Because now, when the actuary requests capacity to calculate 1,000 scenarios, the provider can allocate to her more than she got last time.
The following table lays out the essential parts for comparison. In the first two columns we have the “fixed technology” setup: capacity was defined according to peak usage and set up to just barely satisfy requirements.
The last two columns show how it would look using scalable technology. All of this is assuming that we’re running our process beginning April 1, covering the quarter which ended on March 31. [Just for the example’s sake, we’ll also assume that this April 1 is a Monday.]
Using Fixed Technology Baseline | Using Fixed Technology 10x requirement | Using Scalable Cloud Technology Baseline | Using Scalable Cloud Technology 10x requirement | |
# Scenarios / use case | 10 | 100 | 10 | 100 |
# use cases | 10 | 10 | 10 | 10 |
Total # of Scenarios | 100 | 1,000 | 100 | 1,000 |
Time per scenario | 2 hours | 2 hours | 2 hours | 2 hours |
Initial Computing Hours | 200 | 2,000 | 200 | 2,000 |
Factor to distribute workload and store results | 1.0 | 1.0 | 1.1 | 1.2 |
Total Computing Hours | 200 | 2,000 | 240 | 2,400 |
# of Cores Allocated | 4 | 4 | 100 | 1,000 |
# of calendar Hours to complete calculations | 50 | 500 | 2.2 | 2.4 |
Results available (from Tuesday at 6 AM, BD 2) | Thursday, 8 AM Business day 4 | Tuesday, 4 AM Business day 23 | Tuesday, 8:15 AM Business day 2 | Tuesday, 8:30 AM Business day 2 |
Note – the factor for distributing workload and gathering results applies and increases the time slightly using scalable computing. This amount is hypothetical, but does represent historical experience.
There are two very relevant comparisons to be made: fixed setup versus scalable setup in columns 1 and 3. Under the fixed setup, you get initial results after 2 days. Under the scalable setup, you get results after 2 hours.
And the second comparison is between columns 2 and 4. Using that old fixed setup, it takes about 3 weeks to get results. Using scalable setup? Still just a little over 2 hours. Now that’s what we call seeing the future.
What if the company wants more? Maybe more sensitivity tests? Or a re-run with a different assumption set, to see how results might be impacted? If that’s not being done using scalable technology, get ready to wait.
Each of those situations is a set up for additional delays. Because power is always limited to the capacity available, the speed with which those results can be produced is also limited.
We’re not just making this up
One of our clients recently discovered the benefits of having this on-demand capacity. After running an average of 25 scenarios per month in the latter part of 2020, analysis needs spiked in January. A lot. This client kicked off over 700 scenarios in that month alone. In addition, during just the first 8 days of February, there were over 900 scenarios initiated and completed.
That’s over 1,600 scenarios in just 40 days, or more than 40 / day.
Here’s where the rubber meets the road. Each of these scenarios averaged around 24 hours of core run-time. (A “core hour” of run-time means that one core was calculating for one hour).
In order to get those same results under a static capacity setup, that client would have needed to purchase enough to support 1,000 core hours every day.
Which means you need over 40 cores running constantly, for 40 full days, to get those same results in this time frame.
Want to take a guess how many core hours were used per day outside of this peak time? If you said less than 2 per day, you’re right! If our user had bought enough capacity to handle the peak load, during the rest of the year they’d have about 99.8% of that capacity sitting around unused.
That’s a lot of computing power that goes to waste. And don’t forget, there’s also the maintenance expense, initial set-up from the IT department, and, depending on your contract, you might have additional charges to store those results as well.
Oof.
How the cloud makes this possible
So how does all this work in a cloud-based environment? The best example we can think of is what we do here at Slope. When a projection is initiated, we look at how many different scenarios are being evaluated. We allocate machines from our huge bank of availability directly to those runs, specifically for that purpose.
We assign each scenario to a different machine, let it calculate, and then aggregate all the results back together again. [That assignment / aggregation is what adds that slight uptick factor of 1.1 or 1.2 above.] Then we release that machine back into the pool of availability for other clients to use.
Multiple projections can be initiated at the same time, creating demand for additional computing resources. Under a traditional setup, what usually happens is that sequential processing requirements create a queueing situation: One projection goes first, processing until all the required calculations are complete across all scenarios, then the next one goes, and the next one. Each patiently waits its turn on the calculation engine.
Ultimately this takes 10 cycles to get everything done (base case + 9 sensitivities). Could you get it 10x as fast? Under a traditional setup, not without spending 10x as much.
With a scalable setup, however, you can. That’s because you can set up all 10 use cases as their own projection and have them all kick off at the same time, each one grabbing its own miniature block of 10 machines for calculation. So you can get not just 10 scenarios in parallel, but 100 (10×10).
And this is the kind of scalability that can really accelerate your business decision-making. Because now, it’s like you’ve just reached through space-time, grabbed the results that you used to wait days or weeks for, and brought them back into the present.
Which is, you have to admit, kind of like … seeing the future.