
It’s no surprise to the modern actuary that no projection is ever 100% perfect. Every single model-to-actual comparison is going to result in some kind of deviation.

There are plenty of reasons for this, not the least of them being that there are about a billion assumptions that go into every actuarial model. From expenses to commissions to interest credited, actuarial models are just these massive accumulations of potential deviation.
And then we get into comparing results between two systems, which happens when you’re making a conversion from one system to another.
[Quick aside – if you’re considering a model conversion project, check out our 7-Step Guide to Effective Actuarial Model Conversions. It provides good advice and tips for every stage of the journey.]
Even when all of the assumptions and input files are the same between two systems, you can still have different results. Why?
Well, one of the reasons is because of timing. You know, that thing that’s like 90% of comedy.
It shows up in actuarial models when systems have different conventions between them.
Why is timing an issue?
Timing issues are one those little concerns that arise when you have to create a model and make some simplifying assumptions. One assumption most systems use is to assume 12 months in a year, and 30 days in those months. Which, as we all know, is a bit different from the actual calendar of 28, 29, 30, or 31 days in a month.
Why do these simplifications exist? Frankly, it’s because performing daily calculations of all the individual policy cash flows has historically been too calculation-intensive. In order to get results in a reasonable time frame, sacrifices must be made. There are trade-offs to be made between precision of the calculations, compute time, model parsimony, and ease of understanding formulas.
Simplifications may lead to differences in values between two systems, especially if you’re comparing across different conventions.
Some systems might be coded to have all policy value transactions occur at the beginning of the month, and all interest-related transactions occur at the end of the month.
Another may assume everything in the middle of the month, just to simplify the processing and try to minimize errors.
Yet another convention might be to track the daily values of interest accruals, expense charges, mortality and lapses, and so on.
Do note, none of these are necessarily wrong, they’re just different.
How might this show up?
Well, if you’ve assumed policies are issued at the beginning of the month, and the policy was actually issued on the 30th, your policyholder is approximately 1/12 of a year younger than you’ve assumed.
This could impact older ages (high mortality) and high lapse scenarios, because a month could make a pretty big difference.
Another place where model system timing issues may show up is in comparing model values to actual values, especially early in a policy lifetime. A question the actuary should ask, then, upon seeing some deviation between model values and actual values is: Is this a system convention or a model error?
And what’s the difference between those two?
System Convention versus Model Error
A system convention is simply two alternative ways of looking at something. It’s kind of like viewing a sculpture from two different perspectives. Check out this 3-D sculpture on the streets of Paris:
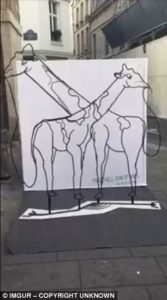
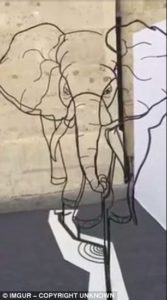
Check out the video here (YouTube).
Depending on how you’re viewing it, you’re not wrong to interpret it as either an elephant or a giraffe. Or both!
In the same way, if you have different system conventions between two actuarial models, you can understand the relationship between the two and it will remain stable over time. If necessary, you could correct for it.
A model error means that something is wrong and should be changed. The difference will grow over time and will impact decision-making in the future, once they are material enough. No matter how you look at it, modeling errors aren’t going to go away. In fact, they may grow to be bigger and more material as you go along.
Let’s illustrate the difference with two examples
[Yay! We get to play with numbers again! This makes the actuary in me happy. :-)]
For the first, let’s suppose we have a deposit to a traditional fixed-interest annuity of $100,000 on the 15th of the month. At the end of the month, the actuary is tasked with determining the reserve value, which is going to be floored at the Account Value at the valuation date.
But which Account Value do you use? If you input this into a model which assumes first-of-the-month transactions, it will assume the $100,000 was deposited on the 1st of the month, and registers $100,304. But the Actual Account value is $100,160. A difference of $144..
Is that wrong? Well, no. Both are right, in the same way that Schroedinger’s cat is both alive and dead.
The admin system is right, in that it tells what the actual balances really are. There really was $100,000 deposited on the 15th. There really was interest that accrued for 15 days, and there really is $100,150 in the account value by the 30th.
And at the same time, the modeling system is right, in that if there is a $100,000 deposit on the 1st, it will grow to $100,310 by the 31st.
It may be different, but it doesn’t mean it’s wrong. It’s just different.
We can see that this error continues at about the same magnitude throughout the projection:
And if you were to compare one full year between the actual value and the model, you’d find pretty tight alignment:
So this is an example of a structural issue that really isn’t an issue at all. Timing differences between the model and actual values will work themselves out over time, and at any specific point in time, they just won’t be that big in the grand scheme of things.
Here’s the other example. Suppose there really was an actual value of $100,000 deposited into the account on the 1st of January, and there was an incorrect value in the admin download of the interest rate to be credited in the model. Instead of 3.65%, suppose it was incorrectly coded as 2.65%.
Now, the value isn’t that different at the first monthly valuation date.
It’s only $61 off, for goodness sake! Might even be small enough that it wouldn’t get flagged as an error.
But over time, the difference grows, to the point where a correction would be warranted and would be justified in spending the time to understand where the error lies.
In this case, it’s clear that there’s an error, because the divergence grows and doesn’t resolve itself at some point.
In some other cases, it might not be so evident, but still, timing issues between systems (especially around cash flows within a specific month) arise during model conversions.
And sometimes actuaries get distracted in searching out and quantifying structural differences that probably aren’t material. [To avoid that, we suggest starting with some prioritization of your conversion structure. Here’s a good discussion about that.]
This keeps you from making progress towards your goals, and getting your work done on-time and with the level of quality you know you can deliver.
So how can you move forward if your model values don’t match what you feel they should?
Maybe it’s that model-to-actual difference and you’re wondering whether it’s material. Or maybe it’s that model-to-model difference and you’re wondering whether it’s an error. Here are 3 steps you should remember to take as you investigate issues like these..
Step 1 – Dive in and see if you can identify whether the difference is structural or a true model error.
If it’s structural, it is going to be evident across all the policies in the model. It will show up as a consistent difference (generally a percentage) of some reference value. When you see consistent deviations from what you expected, both in magnitude and direction, across many policies, there’s a good chance you’re looking at a structural difference.
When deviations don’t fit a pattern, or if there are some policies that are right on while others throw off significant deviations, it’s more likely this is an error somewhere. If it’s an error, that could be anything from a transposed value in a table to a piece of missing information to a bad data call. What you’re looking for is elements that don’t seem to align or that grow bigger over time.
Step 1.a – If it is an error, absolutely correct it. There’s no reason not to. Once you’ve corrected it, you may need to check for additional structural differences. If there are none, proceed to Step 3. If you do discover structural differences, proceed to Step 2.
Step 2 – Decide whether the structural difference is material enough to warrant an adaptation.
The issue of materiality is for another article, one that we absolutely have planned. But for now, you can check out the AAA’s discussion paper on materiality and this definition from an auditor’s perspective.
If you’ve determined that the difference is material enough to make a change, now you’ve got another question to answer. Either you will need to change something about the model or make an after-model adjustment.
Be careful how you do this, though. Your work-around could easily lead to a spiral of unconstrained model adjustments.
For instance, in the above example, you could consider adding on an additional ½ month’s worth of interest to the admin output to account for the uncounted interest. Or you could make a model change to correct for ½ month less interest accrued during the first policy month. [Those are just two examples. Surely actuaries could determine some other solutions.]
Either way, remember that you’re adding something external to your system to create official model results. Your work-around is now part of the model and should be peer reviewed, approved, and moved to production according to your standard model governance framework.
Step 3 – Document what and why of your change.
This applies for both structural and model error changes. Obviously for the model error changes, documentation proves your case and creates the audit trail that helps later reviewers agree that what you did was appropriate. Most actuaries are pretty good at that.
In our experience, more actuaries need to improve documentation practices around systemic differences they’ve uncovered and accounted for. If you (as an actuary) learn something about the modeling system, it is good practice to pass that knowledge on to those who may be encountering the same thing currently, as well as those who come after you.
Good documentation will help that knowledge transfer. And as a result, other members of your organization don’t end up repeating a similar investigation later.
CONCLUSION
Timing issues within an actuarial model often lead to value differences when comparing against other models or actual values. These can masquerade as errors when they are, in fact, structural differences. Understanding which is which will allow actuaries to correctly define what needs to be done, do it, document the solution, and move forward.